Snowflake, What The Mesh?! Featuring James Anderson
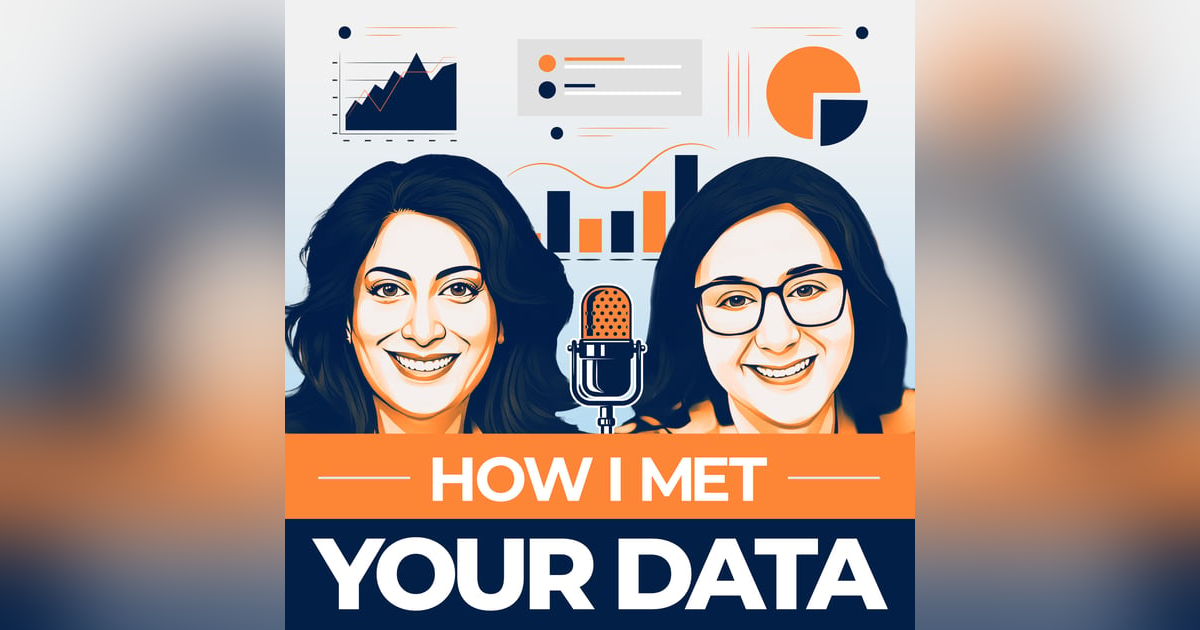
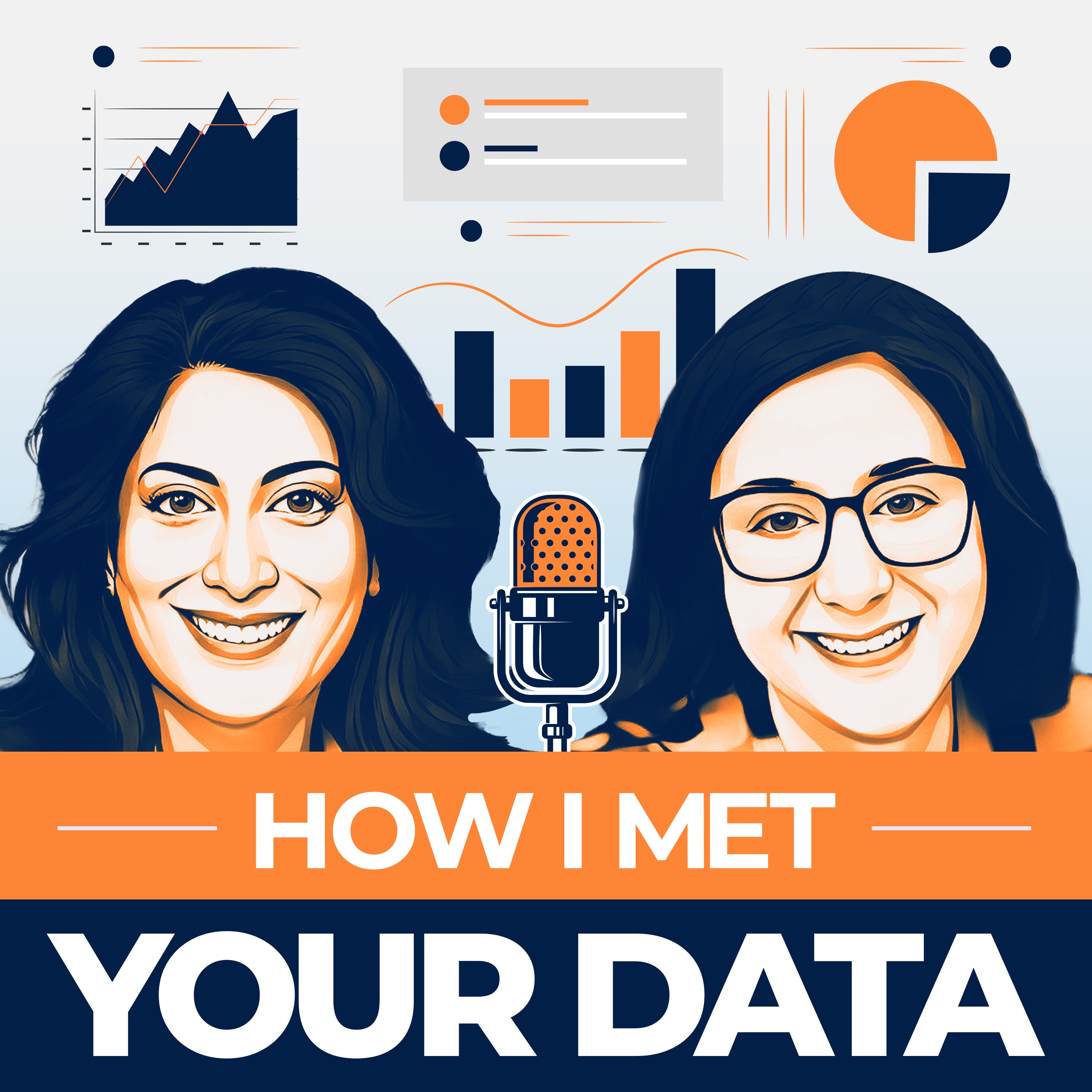
In this episode of How I Met Your Data, we welcome James Anderson, ex-consultant and current Director of Cloud Data Architecture at Snowflake. Hosts Sandy Estrada and Anjali Bansal engage in a comprehensive discussion covering Snowflake's advanced ca...
In this episode of How I Met Your Data, we welcome James Anderson, ex-consultant and current Director of Cloud Data Architecture at Snowflake. Hosts Sandy Estrada and Anjali Bansal engage in a comprehensive discussion covering Snowflake's advanced capabilities, the intricacies of dashboards, and the broader future of data work.
The conversation covers a lot of ground from the critical importance of aligning business goals with data initiatives, the value of Return on Investment (ROI), and the creation of data products. We discuss the dangers of focusing too narrowly on specific use-cases, the benefits of reusability in data products, and the essential roles of assets and collaboration in data analytics. We also debate the often misunderstood concepts of data mesh and the pivotal role of data governance.
The dialogue then extends into Generative AI and its influence on business strategies. We discuss the modern data stack's tool selection process, how customer feedback influences Snowflake's developments, and generative AI's effects on data teams. Additionally, we explore the role of Python in data engineering and then take a stance over the longevity of operational dashboards.
This episode is jam packed with industry trends, practical examples, and thought-provoking insights into data management, governance, AI, and advanced analytics. You are not going to want to miss this one.
00:00 - Welcome and Introductions
01:06 - Funny Studio Moments
01:40 - Catching Up with James Anderson
02:05 - James Anderson’s Career Journey
04:09 - Snowflake’s Data Cloud Architecture
07:09 - Trends in Data Analytics
08:51 - Dive into Generative AI
09:53 - Thoughts on Data Mesh
14:21 - Importance of Data Governance
14:46 - Empowering Innovation with Governance
15:30 - Governance as an Enabler
19:43 - Snowflake’s Shift to Advanced Analytics
20:36 - Customer-Centric Decision Making
25:39 - Impact of Gen AI on Work
28:11 - Adapting to Changing Data Solutions
31:37 - Evolution of Data Interactions
36:14 - Parting Advice: Learn Python
00:00:00,017 --> 00:00:05,017
Welcome back. Sandy here, and I have some good news and some bad news to share about this episode.
2
00:00:05,257 --> 00:00:09,817
The good news is we have a guest joining us. The bad news is he's a vendor.
3
00:00:09,977 --> 00:00:14,137
But all jokes aside, Anjali and I had a fantastic time chatting with James Anderson.
4
00:00:14,457 --> 00:00:18,297
He's an ex-consultant and current director of cloud data architecture at Snowflake.
5
00:00:18,437 --> 00:00:22,657
James and I go way back, and I've always enjoyed our spirited debates,
6
00:00:22,937 --> 00:00:27,097
which is exactly why Anjali and I decided to invite him to the podcast. guests.
7
00:00:27,137 --> 00:00:29,937
We couldn't think of a livelier guest to kick things off with.
8
00:00:30,077 --> 00:00:34,457
Yes, we start by discussing Snowflake, of course, but then we move into other
9
00:00:34,457 --> 00:00:38,877
topics like data mesh, changing dynamics of dashboard usage and data analytics.
10
00:00:39,137 --> 00:00:43,477
And we have a quick debate on the future of data work. We hope you enjoy listening
11
00:00:43,477 --> 00:00:46,417
to this discussion as much as we enjoyed having it. So let's get started.
12
00:00:46,320 --> 00:01:05,840
Music.
13
00:01:06,163 --> 00:01:11,163
Nice. You know when your recording's on and you have the little red flashing
14
00:01:11,163 --> 00:01:13,403
light back in the studio back in the day? Uh-huh.
15
00:01:14,443 --> 00:01:17,103
This is outrageous. And I like that
16
00:01:17,103 --> 00:01:19,843
we're recording now, this part of this conversation. It makes me happy.
17
00:01:21,343 --> 00:01:24,363
I hope this makes it into the podcast. You said I didn't hit the button earlier. That's what I'm...
18
00:01:26,783 --> 00:01:29,703
All right. Are you mentally prepared? You want to get into this?
19
00:01:30,143 --> 00:01:32,703
Am I mentally prepared? I was on a red-eye flight last night.
20
00:01:32,783 --> 00:01:35,303
I'm never mentally prepared. So let's just... Let's roll.
21
00:01:35,683 --> 00:01:40,743
Red Eye. Where did you come from? San Francisco. I've been flying around a lot. Back and forth to HQ.
22
00:01:40,983 --> 00:01:44,243
So James, I've known you for a while now.
23
00:01:44,543 --> 00:01:49,623
For a long while. I don't even know how long. But how about you? Almost 10 years.
24
00:01:49,843 --> 00:01:52,903
I've been at Cervelo for 11. So that's kind of incredible.
25
00:01:53,103 --> 00:01:58,883
Now that I think about it. I started working at Cervelo in November of 2014.
26
00:01:59,403 --> 00:02:02,243
I didn't realize that for some reason. But why don't you introduce yourself
27
00:02:02,243 --> 00:02:05,183
to Anjali and our listeners while you're at it? Sure.
28
00:02:05,443 --> 00:02:09,943
Yeah. So my name is James Anderson. I used to work with Sandy and the team at
29
00:02:09,943 --> 00:02:12,863
Cervelo for I think it was about two years I was there.
30
00:02:12,983 --> 00:02:15,243
I then moved on to another consulting firm.
31
00:02:15,363 --> 00:02:18,063
You know, I started my career at Cervelo as a burst developer.
32
00:02:18,303 --> 00:02:24,183
I was a front end front end dashboard guy building very beautiful dashboards in burst as one one does.
33
00:02:24,343 --> 00:02:29,583
And then as I moved in through my consulting career, I moved myself down the stack.
34
00:02:29,723 --> 00:02:34,023
And by the time I left the consulting space, I was running the platform architecture
35
00:02:34,023 --> 00:02:38,883
team for the firm I was at focused on how do you build and deploy large scale
36
00:02:38,883 --> 00:02:40,603
enterprise wide data platforms?
37
00:02:40,763 --> 00:02:43,243
And how do you fit that into a broader enterprise architecture,
38
00:02:43,483 --> 00:02:48,043
I left consulting in 2020, right at the height of the pandemic moved over to
39
00:02:48,043 --> 00:02:51,163
snowflake because I had been doing almost exclusively snowflake implementations
40
00:02:51,163 --> 00:02:54,623
for at least the previous four years before that.
41
00:02:54,703 --> 00:02:57,443
So I've been in and around the snowflake ecosystem for a long time.
42
00:02:57,543 --> 00:03:00,903
And I have been at snowflake now for just shy of four years.
43
00:03:01,183 --> 00:03:04,703
For the first couple of years, I ran a number of different sales engineering
44
00:03:04,703 --> 00:03:08,603
teams focused on first large accounts in the greater New England area.
45
00:03:08,803 --> 00:03:12,143
And then for the last two years, I had been running the sales engineering team
46
00:03:12,143 --> 00:03:14,663
focused on large life sciences organizations.
47
00:03:15,083 --> 00:03:19,423
So pharmaceutical, medical device, medical distribution, CRO.
48
00:03:19,643 --> 00:03:24,823
And starting February, I moved into our new data cloud architecture team focused
49
00:03:24,823 --> 00:03:28,743
on how do you fit Snowflake into to a more broad enterprise architecture.
50
00:03:29,023 --> 00:03:33,743
That's my complete end-to-end life that I have had in the data space at this
51
00:03:33,743 --> 00:03:35,663
point. What do you do outside of the data space?
52
00:03:35,883 --> 00:03:40,703
What kind of hobbies or interests do you have? Or do you work so hard you have no hobbies, interests?
53
00:03:40,803 --> 00:03:46,043
Well, in the last month or so, let's see, I've been on 10 planes in the last 20 days.
54
00:03:46,243 --> 00:03:49,943
So I don't really have time for other things outside of that right this second.
55
00:03:50,223 --> 00:03:53,723
In general, I have two young children who I adore.
56
00:03:54,314 --> 00:03:58,314
Immensely and spend most of my time entertaining or finding ways to entertain.
57
00:03:58,514 --> 00:04:02,594
I also like to play golf like any good data executive likes to do.
58
00:04:02,794 --> 00:04:05,874
Those are my main hobbies if I have time for those things.
59
00:04:06,214 --> 00:04:09,754
And we'll see if that ever comes back, if I ever get more time for that.
60
00:04:09,994 --> 00:04:13,134
So is that a newly formed team at Snowflake? Yeah.
61
00:04:13,194 --> 00:04:17,434
So historically, when our customers had come to us and said,
62
00:04:17,514 --> 00:04:20,194
hey, what is our recommended architectural approach?
63
00:04:20,734 --> 00:04:24,754
Hey, should we run a data warehouse? Should we transition to to being more of
64
00:04:24,754 --> 00:04:26,134
a data lake type of construct?
65
00:04:26,954 --> 00:04:31,354
What do you guys think about data fabric? Definitely getting a lot of people asking about data mesh.
66
00:04:31,594 --> 00:04:34,694
In the past, our answer was, oh, you can do anything you want.
67
00:04:34,814 --> 00:04:40,154
As an organization, we're really trying to get our customers to think about
68
00:04:40,154 --> 00:04:44,794
Snowflake, not just as a cloud data warehouse or cloud data lake or something
69
00:04:44,794 --> 00:04:48,494
of that nature, but really understand that this is a full-blown end-to-end data platform.
70
00:04:48,674 --> 00:04:53,554
And so we've built an architectural framework that we call the data cloud architecture,
71
00:04:53,874 --> 00:04:59,254
to help our customers who want to truly adopt Snowflake as a platform and get
72
00:04:59,254 --> 00:05:01,514
better value out of the data that they have.
73
00:05:01,714 --> 00:05:07,614
A lot of this framework is around how do you tie value to what you're building?
74
00:05:07,754 --> 00:05:11,574
And how do you treat the things that you're building not as tools for decision
75
00:05:11,574 --> 00:05:15,654
making, but instead as an asset that has a measurable ROI?
76
00:05:16,094 --> 00:05:21,654
In the past, when a client says, says, hey, where does this fit in my larger architecture?
77
00:05:22,134 --> 00:05:29,174
The answer would naturally be go to consultant X who's worked across a lot of technologies, right?
78
00:05:29,354 --> 00:05:33,574
To help you pull that together. So now it seems like Snowflake's building a
79
00:05:33,574 --> 00:05:36,054
team that has an opinion, which is fine.
80
00:05:36,214 --> 00:05:40,594
Find it interesting to see how clients react to that because you're still getting
81
00:05:40,594 --> 00:05:42,774
the advice from the vendor at the end of the day.
82
00:05:42,854 --> 00:05:46,494
Yeah, so one of the things that I tell customers from the jump.
83
00:05:46,842 --> 00:05:52,662
Is that my team is certainly going to look at this from a snowflake first perspective,
84
00:05:52,902 --> 00:05:55,862
but we're not going to look at it from a snowflake only perspective.
85
00:05:56,022 --> 00:06:01,602
We are a little bit trying to provide some level of free consulting to help lay that foundation.
86
00:06:01,782 --> 00:06:04,602
Now, we're not going to then turn around and do your whole implementation for
87
00:06:04,602 --> 00:06:06,562
free for you for all the way through, right?
88
00:06:06,642 --> 00:06:11,682
We're going to rely on our partners like Cervelo to actually take this sort
89
00:06:11,682 --> 00:06:14,362
of architectural vision and implement it and put it in place.
90
00:06:14,442 --> 00:06:17,962
Because there's a lot, It's not just a technical underpinnings, right?
91
00:06:18,022 --> 00:06:22,282
There's a shift in how you think, and there's a change management process that
92
00:06:22,282 --> 00:06:23,882
has to be taken into account.
93
00:06:24,182 --> 00:06:29,342
So as you're talking about this, I guess because Snowflake made this shift,
94
00:06:29,662 --> 00:06:32,162
it sounds like that's been a big trend, right?
95
00:06:32,262 --> 00:06:37,682
Clients really trying to figure out not just what is this technology itself
96
00:06:37,682 --> 00:06:43,122
in isolation, but how does it interplay with all the other things I'm trying to do in my stack?
97
00:06:43,122 --> 00:06:47,582
The question you're trying to answer for them is, here are the other pieces
98
00:06:47,582 --> 00:06:49,662
of my stack. Here's the other things I'm trying to achieve.
99
00:06:50,002 --> 00:06:56,902
Help me put this wonderful modular puzzle together that has been created as
100
00:06:56,902 --> 00:06:59,882
the modern, quote unquote, modern stack.
101
00:07:00,002 --> 00:07:02,602
It's modular, right? That's usually what people push for.
102
00:07:02,882 --> 00:07:08,722
I know companies are moving in certain directions, but that's typically what people push for today.
103
00:07:09,022 --> 00:07:11,962
Are there any other trends that you're seeing out there? I mean,
104
00:07:12,042 --> 00:07:15,762
data mesh is the most popular conversation that we've had.
105
00:07:15,882 --> 00:07:19,022
I mean, is it not the most popular conversation you've had in the last year
106
00:07:19,022 --> 00:07:20,262
and a half? Like, really? No.
107
00:07:20,782 --> 00:07:25,962
Really? Yeah. That's surprising, because the number of customers that have come
108
00:07:25,962 --> 00:07:31,042
to me and said, Hey, I have this partner who's really pushing the data mesh paradigm.
109
00:07:31,462 --> 00:07:35,142
What do you guys think? And how can Snowflake support this data mesh strategy?
110
00:07:35,402 --> 00:07:40,702
Right? Is outrageous. I think last summer I had this conversation with more
111
00:07:40,702 --> 00:07:42,282
than half of the customers that I supported.
112
00:07:42,382 --> 00:07:48,882
Everybody likes this idea of domain ownership and putting the capabilities and
113
00:07:48,882 --> 00:07:52,162
the infrastructure in the hands of the people who actually understand the data.
114
00:07:52,162 --> 00:07:56,262
On the flip side, when it comes to a true data mesh, the idea of domain ownership
115
00:07:56,262 --> 00:08:01,782
includes the idea that you have engineers inside of each domain who will take
116
00:08:01,782 --> 00:08:05,662
who will ingest process and create these data products.
117
00:08:05,862 --> 00:08:09,422
And I have a whole beef on the term data product, which we can get into later.
118
00:08:09,582 --> 00:08:14,562
But there's a level of sort of organizational change that goes into how you
119
00:08:14,562 --> 00:08:17,242
staff. Yeah, or how you organize period, right.
120
00:08:17,322 --> 00:08:20,262
So from our frameworks perspective, from this sort of data cloud architecture
121
00:08:20,262 --> 00:08:23,842
perspective, we're taking a lot of the positives of data mesh in terms of,
122
00:08:23,842 --> 00:08:27,502
we want to enable that free flow of data and applications across your ecosystem,
123
00:08:27,682 --> 00:08:32,162
we want to make it easier for you to collaborate with your business and with your partners.
124
00:08:32,763 --> 00:08:36,423
And with your customers even, but not do it in a way where you have to actually
125
00:08:36,423 --> 00:08:37,803
transform how you run your business.
126
00:08:37,943 --> 00:08:40,583
And I understand that there are consulting firms out there who specialize in
127
00:08:40,583 --> 00:08:41,243
business transformation.
128
00:08:41,503 --> 00:08:45,423
So this is very appealing to them, because there's a huge project that comes behind this.
129
00:08:45,783 --> 00:08:49,423
So I think that's one. The other is obviously generative AI and everything around
130
00:08:49,423 --> 00:08:50,783
the whole generative AI space.
131
00:08:51,063 --> 00:08:54,503
And I know when I was listening to your first episode, I was texting Sandy with
132
00:08:54,503 --> 00:08:56,343
all sorts of opinions on this.
133
00:08:56,483 --> 00:08:59,783
Well, that's how you ended up on the podcast. You were texting me right after
134
00:08:59,783 --> 00:09:03,983
our first episode, So you're sending me these rant texts about Gen AI.
135
00:09:04,203 --> 00:09:07,083
And I was like, wow, we got to get him on. He's ready to go.
136
00:09:07,543 --> 00:09:11,723
I was live texting you as I was listening with my live feedback. Yes.
137
00:09:12,463 --> 00:09:15,163
But would you expect me to do it any other way, Sandy? I mean,
138
00:09:15,183 --> 00:09:17,283
come on. No, absolutely not. That's what I love about you, James.
139
00:09:17,383 --> 00:09:21,943
I think when it comes to data mesh, yes, we do have clients who are thinking about it.
140
00:09:22,003 --> 00:09:25,103
We don't push it as a consulting firm. And I think because we know,
141
00:09:25,203 --> 00:09:29,763
we know what it takes. And when I can't get a company to even think about how
142
00:09:29,763 --> 00:09:34,643
to model data for consumption, why am I going to start pushing a concept that they're not ready for?
143
00:09:34,743 --> 00:09:37,043
As a consultant, I'm not even going to talk about our company,
144
00:09:37,163 --> 00:09:40,163
but as a consultant, I personally have a challenge with that.
145
00:09:40,443 --> 00:09:45,963
With the push of new concepts to clients when they are not mature enough to
146
00:09:45,963 --> 00:09:49,083
get to that space, that's something that I holistically will not do.
147
00:09:49,403 --> 00:09:53,063
I want people to be successful in what they're trying to achieve.
148
00:09:53,223 --> 00:09:56,403
That's my goal. So yeah, I don't push it personally.
149
00:09:56,583 --> 00:10:00,683
I think data products, I know you don't like that term. I don't like many terms
150
00:10:00,683 --> 00:10:03,583
because people define things however they want to define them.
151
00:10:03,943 --> 00:10:07,723
So I always say, you have your definition, I have mine. Let's just make sure
152
00:10:07,723 --> 00:10:10,283
we all understand where we're coming from is kind of my goal in life.
153
00:10:10,703 --> 00:10:14,643
But data mesh, yeah, we have clients have talked about it.
154
00:10:14,723 --> 00:10:18,763
And most of the time, they're looking at it as a tooling question.
155
00:10:19,183 --> 00:10:23,683
What are the technical pieces I need to create a quote unquote data mesh? And I tell them,
156
00:10:24,085 --> 00:10:28,085
That's nice. That's not going to solve your problem. Well, yeah,
157
00:10:28,265 --> 00:10:32,305
I mean, I agree with the maturity aspect, but there are companies out there
158
00:10:32,305 --> 00:10:33,805
who are structured in this way.
159
00:10:34,065 --> 00:10:37,925
Extremely large e-commerce retailer here in the Boston area is structured that
160
00:10:37,925 --> 00:10:41,285
way, right? That's how their business is set up.
161
00:10:41,385 --> 00:10:43,665
So fine, you know what? That makes sense.
162
00:10:43,865 --> 00:10:47,185
Good for you. Good job. For everybody else, yikes.
163
00:10:48,685 --> 00:10:52,465
So my BeatBoot data product, in my opinion, a data product is something that
164
00:10:52,465 --> 00:10:54,545
you build in order to sell to the rest of your mesh.
165
00:10:54,725 --> 00:10:58,185
You don't know that anybody's going to use it. You just think that it is.
166
00:10:58,225 --> 00:11:02,305
But you have to like spend cycles building it in such a way that it feels like
167
00:11:02,305 --> 00:11:06,645
an actual product, which in my mind actually lowers the ROI that you might get
168
00:11:06,645 --> 00:11:09,585
out of that because your input costs are higher.
169
00:11:09,705 --> 00:11:13,285
Your resource costs are higher. The technology costs that you're using in order
170
00:11:13,285 --> 00:11:15,345
to make this thing look like a product are probably higher.
171
00:11:15,465 --> 00:11:18,785
And you could probably do something with lower input costs and get the same
172
00:11:18,785 --> 00:11:20,065
level of adoption across the board.
173
00:11:20,165 --> 00:11:24,865
That's why we're really focused on this idea of assets sets and tying everything back to ROI.
174
00:11:25,085 --> 00:11:28,025
Because then it's really about are you building the right things?
175
00:11:28,285 --> 00:11:32,065
Like previous consulting firm, we did a project for a large hospital network
176
00:11:32,065 --> 00:11:33,905
in general Massachusetts area.
177
00:11:34,225 --> 00:11:37,965
And we built them a dashboard. It was there was a lot of data that came into
178
00:11:37,965 --> 00:11:40,825
this and it was a pretty hefty project and program that we did.
179
00:11:41,065 --> 00:11:45,025
It was a bed management dashboard where they would be able to see sort of the
180
00:11:45,025 --> 00:11:48,485
layout of how many beds were available and so on and so forth,
181
00:11:48,605 --> 00:11:53,325
and some level of a sort of a light forecast against sort of where the beds
182
00:11:53,325 --> 00:11:56,845
would be, so that they could do their staffing in such a way that they saved
183
00:11:56,845 --> 00:12:01,405
something, they told us they saved something in the realm of like $70 million in staffing costs.
184
00:12:01,525 --> 00:12:05,625
And the fact that they like made an effort to track that number tells me that
185
00:12:05,625 --> 00:12:08,865
they actually understood the ROI that they were going for.
186
00:12:09,025 --> 00:12:12,805
Yeah, I think I look at it similar, but different than the approach you just
187
00:12:12,805 --> 00:12:16,445
laid out, right? I guess, James, like the example you gave, right? It was a.
188
00:12:16,919 --> 00:12:21,799
There was a specific ROI that we were targeting, a very pointed product that was being created.
189
00:12:21,999 --> 00:12:25,739
I look at something like that. I'm like, all right, that's the goal of the business.
190
00:12:25,779 --> 00:12:27,359
That's your business strategy, right?
191
00:12:27,619 --> 00:12:29,639
And ultimately, there's a product that's tied to that.
192
00:12:29,919 --> 00:12:32,999
But I look at all the data elements that are tied to that product.
193
00:12:33,079 --> 00:12:36,599
And I think to myself, this concept of, because this is what happens in organizations,
194
00:12:36,879 --> 00:12:39,559
right? They have this ROI item that they're trying to achieve.
195
00:12:39,879 --> 00:12:42,599
And they're like, all right, we need to get all this work done for this one
196
00:12:42,599 --> 00:12:47,919
use case. And then all the work that is done for that use case is only for that use case.
197
00:12:47,999 --> 00:12:51,659
Everything around it gets orphaned. That big data product probably includes
198
00:12:51,659 --> 00:12:56,559
20 others underneath it, pieces and components of that product that are very
199
00:12:56,559 --> 00:12:59,859
specific to domains that other people can re-leverage.
200
00:12:59,859 --> 00:13:04,539
So you need to distill down what you're actually trying to do into components
201
00:13:04,539 --> 00:13:06,959
so that people can re leverage that information.
202
00:13:07,119 --> 00:13:11,539
And then the ROI goes way up. And not only for that one project that you just
203
00:13:11,539 --> 00:13:15,819
finished on the bedside, but the reuse of those analytics and those different
204
00:13:15,819 --> 00:13:17,619
data products that made that larger one, right?
205
00:13:17,719 --> 00:13:21,039
That's the way I look at it. But the problem is people don't think that way. Right.
206
00:13:21,199 --> 00:13:25,019
Agreed. And this is why, this is why we're, again, we're focused on assets and
207
00:13:25,019 --> 00:13:29,139
why we're focused on ROI, because to your point, there's 20 different other
208
00:13:29,139 --> 00:13:32,739
things that are part of that, that are part that go into building this,
209
00:13:32,739 --> 00:13:34,759
this more broad, larger asset.
210
00:13:34,859 --> 00:13:38,419
This is, and my issue with data mesh becomes that you end up actually building
211
00:13:38,419 --> 00:13:40,959
in silos and then trying to share at the end. Yeah. Right.
212
00:13:41,279 --> 00:13:45,619
Versus like building in a collaborative fashion is is ultimately how you're
213
00:13:45,619 --> 00:13:47,359
going to drive the most efficiencies across the board.
214
00:13:47,479 --> 00:13:51,939
And so part of our goal as a data cloud architecture team is to help to drive
215
00:13:51,939 --> 00:13:56,659
those efficiencies and help reduce that and drive that collaboration by making
216
00:13:56,659 --> 00:14:00,199
it clear who owns what and what's out there.
217
00:14:00,279 --> 00:14:04,399
Anjali, from a data mesh perspective, have you been hearing anything around
218
00:14:04,399 --> 00:14:08,899
that? I hear a little bit of chatter, but I think it's similar.
219
00:14:09,239 --> 00:14:13,799
Sandy, to the approach that you laid out in terms of.
220
00:14:14,516 --> 00:14:16,396
Our clients aren't ready for
221
00:14:16,396 --> 00:14:21,396
kind of what it takes to be successful with data mesh type of approach.
222
00:14:21,676 --> 00:14:27,356
And one of the key foundations for being successful with the data mesh is having
223
00:14:27,356 --> 00:14:32,836
a highly governed approach to your data, setting up a standard to say,
224
00:14:32,936 --> 00:14:36,596
in order to be considered a data product in our mesh,
225
00:14:36,796 --> 00:14:41,916
you need to meet these handful of criteria and actually track against that.
226
00:14:41,916 --> 00:14:45,876
In the consulting space, I always remember governance being the third rail of a proposal.
227
00:14:46,396 --> 00:14:49,636
Don't put governance in there because nobody's going to buy the project or it's
228
00:14:49,636 --> 00:14:51,176
going to be the first thing that gets pulled out.
229
00:14:51,236 --> 00:14:55,056
MDM and governance were the two things that always got stripped out.
230
00:14:55,176 --> 00:14:57,996
It's just like, I just don't even touch it. Those who toil in the governance
231
00:14:57,996 --> 00:15:01,396
space on the consulting side, I feel the most sympathy for ever.
232
00:15:01,556 --> 00:15:05,536
It's not- You're talking directly to Anjali. I know. That's why I'm talking.
233
00:15:05,796 --> 00:15:10,536
I'm sending this at Anjali and telling her how much, how bad I feel for her
234
00:15:10,536 --> 00:15:12,196
in this particular scenario.
235
00:15:13,096 --> 00:15:19,376
Thank you for that. You know, a lot of our clients are actually struggling with,
236
00:15:19,416 --> 00:15:24,356
you know, with data challenges and have opened up their eyes to the need for
237
00:15:24,356 --> 00:15:30,036
finding accountability and focusing on the confidence and fidelity of their data.
238
00:15:30,096 --> 00:15:34,956
So I think that as we kind of look forward, maybe governance will no longer
239
00:15:34,956 --> 00:15:39,576
be that forgotten forgotten child and really be the golden child of the family.
240
00:15:39,876 --> 00:15:42,816
You know, back to the other topic that's been very popular around generative
241
00:15:42,816 --> 00:15:44,796
AI and everybody needing an AI strategy.
242
00:15:45,016 --> 00:15:50,796
So at our summit last year, Frank Slootman, our former CEO on stage said, you can't have.
243
00:15:51,857 --> 00:15:55,257
An AI strategy without a good data strategy. It doesn't surprise me,
244
00:15:55,357 --> 00:16:00,697
Anjali, that governance is less of a problem and people are looking at it more
245
00:16:00,697 --> 00:16:02,957
because they're being asked to build an AI strategy.
246
00:16:03,217 --> 00:16:08,477
And you can have the best LLMs and the best generative AI capabilities and the
247
00:16:08,477 --> 00:16:09,597
best chat bots in the world.
248
00:16:09,677 --> 00:16:13,137
But if your data quality isn't there, you're training models against trash.
249
00:16:13,357 --> 00:16:15,037
So you're going to get trash back.
250
00:16:15,377 --> 00:16:18,377
The good thing about Gen AI is that it's
251
00:16:18,377 --> 00:16:21,477
highlighted highlighted the fact that good quality sound
252
00:16:21,477 --> 00:16:24,737
data and mastering of metadata is
253
00:16:24,737 --> 00:16:27,537
critical to be able to do something like that right
254
00:16:27,537 --> 00:16:30,597
now everybody wants it because it looks so cool and i'd love
255
00:16:30,597 --> 00:16:33,337
to chat with my data someday hopefully we'll get there
256
00:16:33,337 --> 00:16:36,857
you can't get there if your data is out of quality you can't get there if your
257
00:16:36,857 --> 00:16:41,977
your context around your data is aloof right this is the conversation i had
258
00:16:41,977 --> 00:16:47,097
yesterday with the ceo everybody wants to everybody wants a chatbot And I refuse
259
00:16:47,097 --> 00:16:50,217
because they don't trust the data underneath it. And then they all go off and
260
00:16:50,217 --> 00:16:51,297
build in their own little world.
261
00:16:51,417 --> 00:16:55,877
And, and then there's four different definitions of ACV or whatever it is that
262
00:16:55,877 --> 00:16:59,877
they're looking at and revenue and or, you know, four definitions of churn and none of them are right.
263
00:16:59,957 --> 00:17:03,597
And then when you ask them, well, how do you define that? I would say maybe
264
00:17:03,597 --> 00:17:05,817
not none of them are right. Maybe all of them are right.
265
00:17:05,917 --> 00:17:09,617
It's just a matter of the intent behind it. What's my intent?
266
00:17:09,777 --> 00:17:10,577
What am I trying to drive?
267
00:17:10,777 --> 00:17:15,597
It's intent, it's context, and it's quality of the data asset itself that you're going after.
268
00:17:15,977 --> 00:17:19,817
Ultimately, none of this is going to work if companies don't bring together.
269
00:17:20,337 --> 00:17:25,157
Governance with the data teams and the AI teams and the digital teams into one
270
00:17:25,157 --> 00:17:28,697
package and figure out how do we collaborate to solve these problems.
271
00:17:29,325 --> 00:17:33,145
Because if everybody keeps working in isolation, A, they're only going to have
272
00:17:33,145 --> 00:17:34,145
part of the building block.
273
00:17:34,325 --> 00:17:37,285
No one's going to know how to use it. No one's going to trust it.
274
00:17:37,405 --> 00:17:40,605
And it's just going to be a mess again, except on modern platforms.
275
00:17:41,245 --> 00:17:46,745
Well, you keep saying modern platforms. And this is a conversation I've been having as well,
276
00:17:46,865 --> 00:17:53,725
where people in the sort of mid 2010s started to migrate off of their on-premise
277
00:17:53,725 --> 00:17:58,445
data centers into the cloud with the intent of, oh, we built all these silos on-prem.
278
00:17:58,445 --> 00:18:01,865
We're going to move into the cloud and and the silos are just going to magically disappear.
279
00:18:02,445 --> 00:18:07,405
And they picked up and moved their silos yeah and then they kept being siloed in the cloud so
280
00:18:07,825 --> 00:18:12,605
modern stack doesn't always apply if you're if you're not breaking down the
281
00:18:12,605 --> 00:18:15,965
silos and i think a good and you know back to the whole governance aspect like
282
00:18:15,965 --> 00:18:20,085
a good governance is a double-edged sword here right there is such a thing as
283
00:18:20,085 --> 00:18:24,065
too much governance right you put you make it too hard to participate everybody's
284
00:18:24,065 --> 00:18:26,765
going to go off and build in their own silos and shadow it runs runs rampant.
285
00:18:26,785 --> 00:18:31,305
My whole thing around this modern stack has to be in conjunction with a modern
286
00:18:31,305 --> 00:18:33,765
way of thinking, right? Modern ways of working too.
287
00:18:34,125 --> 00:18:38,405
Yes. A hundred percent. Right. And you can't. Yeah. I mean, you're talking about
288
00:18:38,405 --> 00:18:39,705
governance and I agree with you, right?
289
00:18:39,805 --> 00:18:43,745
I look at this and I know Anjali agrees with me as well is governance should
290
00:18:43,745 --> 00:18:47,345
be focused on outcomes and impact that you're trying to achieve.
291
00:18:47,465 --> 00:18:50,725
At the end of the day, it's an enablement factor, right? What am I trying to
292
00:18:50,725 --> 00:18:55,705
enable and building a process that supports that use case or enablement that
293
00:18:55,705 --> 00:18:59,225
you're trying to go forward with, whether it's mastering of data,
294
00:18:59,505 --> 00:19:02,485
you know, reviewing quality and building a process around that,
295
00:19:02,525 --> 00:19:06,285
or even security and masking policies, etc.
296
00:19:06,545 --> 00:19:10,965
But if you're creating processes that restrain the business from doing things,
297
00:19:11,005 --> 00:19:13,405
then you're doing governance for the sake of governance.
298
00:19:13,485 --> 00:19:16,125
And you're actually, you know, not doing it for the sake of the outcome.
299
00:19:16,285 --> 00:19:19,305
And I think people go one way or the other on that. And it's tough,
300
00:19:19,405 --> 00:19:21,905
because the first conversation people typically have is.
301
00:19:22,303 --> 00:19:24,863
Yeah, what's the organization I need to have for governance?
302
00:19:25,143 --> 00:19:28,703
It's just like, I don't want to answer that question. I actually don't care.
303
00:19:28,863 --> 00:19:32,163
The question I want to answer is what do you need to solve for and put the right
304
00:19:32,163 --> 00:19:33,083
process in place for that?
305
00:19:33,243 --> 00:19:37,683
Yeah, I mean, ultimately, governance needs to be an enabler for innovation.
306
00:19:37,843 --> 00:19:40,043
It cannot be the inhibitor to innovation.
307
00:19:40,363 --> 00:19:43,683
It has to be flexible enough to allow for innovation.
308
00:19:43,943 --> 00:19:47,183
Exactly. One question I had, you know, I've been reading a lot about some of
309
00:19:47,183 --> 00:19:51,123
the bold moves that Snowflake has made around MLOps, LLMs, etc.
310
00:19:51,283 --> 00:19:58,763
You guys have kind of taken a shift into the world of advanced analytics, data science, etc.
311
00:19:58,983 --> 00:20:02,423
So I'm curious, what's the thinking behind that?
312
00:20:02,563 --> 00:20:06,523
And how do you envision that shaping the future of data management?
313
00:20:06,663 --> 00:20:10,203
Because historically, right, and I love this idea, by the way,
314
00:20:10,263 --> 00:20:12,663
because I'm all about bringing these worlds together.
315
00:20:12,763 --> 00:20:16,023
As I just said, these teams have to collaborate, they have to be working on
316
00:20:16,023 --> 00:20:19,623
the same platform, not platform necessarily, but the same data sets,
317
00:20:19,763 --> 00:20:21,323
right, the same data products.
318
00:20:21,963 --> 00:20:27,063
So is it really just a shift in that direction of trying to bring these worlds together?
319
00:20:27,283 --> 00:20:30,683
So I think there's a couple of different answers. And I don't,
320
00:20:30,683 --> 00:20:35,963
I don't promise to speak for our product organization and for our leadership in that way.
321
00:20:36,003 --> 00:20:42,603
But yeah, you know, we've made a lot of strategic acquisitions in the last 18 to 24 months, right?
322
00:20:42,683 --> 00:20:46,523
Companies like Neva, which is where Sridhar, our new CEO came from, right?
323
00:20:46,583 --> 00:20:51,983
And And partnerships as well, where we're trying to, again, be a more robust
324
00:20:51,983 --> 00:20:53,323
platform and bring these capabilities.
325
00:20:53,663 --> 00:20:57,563
But at the end of the day, I have never worked for a company or even when I
326
00:20:57,563 --> 00:21:01,643
was on the partner side, worked with a company who listens to their customers
327
00:21:01,643 --> 00:21:05,543
as much as Snowflake does and makes decisions in that direction.
328
00:21:05,683 --> 00:21:08,763
Many people in the sales organization who have been here for a long time can
329
00:21:08,763 --> 00:21:12,723
point to specific features and then point to the specific customer who asked for it.
330
00:21:12,763 --> 00:21:16,223
And this goes back to things like Snowpipe. You know, data sharing came out
331
00:21:16,223 --> 00:21:18,683
of a very specific ask by one of our largest accounts.
332
00:21:19,103 --> 00:21:22,923
We want to be able to make this data available to our customers.
333
00:21:23,163 --> 00:21:27,703
This shift that you're seeing comes from a desire for our customers,
334
00:21:27,903 --> 00:21:33,243
for some of from some of our largest customers to consolidate from their tooling perspective, right?
335
00:21:33,343 --> 00:21:36,683
You know, you look at that slide that gets published every year of the tools
336
00:21:36,683 --> 00:21:40,003
of the modern data stack, and it just keeps getting bigger, right?
337
00:21:40,063 --> 00:21:41,723
It's just like every tool under
338
00:21:41,723 --> 00:21:44,563
the sun all of a sudden fits under this concept of modern data stack.
339
00:21:44,783 --> 00:21:49,783
And there was a scenario in sort of the early 2020s.
340
00:21:49,783 --> 00:21:52,983
So in the last kind of couple of years where everybody was buying all these
341
00:21:52,983 --> 00:21:57,683
like bespoke tools to do this job, and then it spend was running rampant,
342
00:21:57,683 --> 00:22:01,903
and then the pandemic hits, and then everybody's trying to like, save money.
343
00:22:02,003 --> 00:22:06,463
And they're like, wait a second, why do we own data IQ and data robot?
344
00:22:06,583 --> 00:22:10,203
What is happening here? This doesn't make any sense. So from a Snowflake perspective,
345
00:22:10,343 --> 00:22:13,463
right, for many of our largest customers who have consolidated and brought a
346
00:22:13,463 --> 00:22:14,983
lot of their data estate.
347
00:22:15,552 --> 00:22:19,372
To be stored and processed using Snowflake, they wanted to say,
348
00:22:19,472 --> 00:22:22,612
okay, wait, I want to be able to to code in Python, right?
349
00:22:22,672 --> 00:22:24,572
I want to be able to write data engineering pipelines in Python,
350
00:22:24,692 --> 00:22:25,752
here is Snowpark, right?
351
00:22:25,852 --> 00:22:28,932
So you have this ability now to code in the language that you feel more comfortable
352
00:22:28,932 --> 00:22:30,732
to build those declarative pipelines.
353
00:22:30,912 --> 00:22:35,412
But now my data science teams want to use Snowpark and, and we're not necessarily
354
00:22:35,412 --> 00:22:39,112
optimized, you know, our warehouses aren't necessarily optimized for those types of workloads.
355
00:22:39,312 --> 00:22:42,652
Okay, here comes Snowpark optimized warehouses. And then now we've We've been
356
00:22:42,652 --> 00:22:47,012
building applications. We love using Snowflake to power some of our analytical applications.
357
00:22:47,212 --> 00:22:51,192
We want to be putting more of our applications and consolidating this into Snowflake.
358
00:22:51,292 --> 00:22:52,492
Great. You guys build with containers.
359
00:22:52,912 --> 00:22:57,292
Awesome. Snowpark Container Services. It's very much a feedback loop with our customers.
360
00:22:57,632 --> 00:23:01,112
And yes, all of these things have the word snow in them. And we all have to be okay with that.
361
00:23:02,312 --> 00:23:06,832
They will always. And we're getting over it. Yeah. I think about 1999 moving
362
00:23:06,832 --> 00:23:11,132
forward in the on-prem world, let's say Oracle, right?
363
00:23:11,132 --> 00:23:16,272
The big challenge back then was that Oracle went off and bought all these companies
364
00:23:16,272 --> 00:23:17,412
and tried to integrate it.
365
00:23:17,532 --> 00:23:21,432
And as a consultant, I remember saying to them, yeah, they bought all this stuff,
366
00:23:21,612 --> 00:23:24,232
one tool, but you still have to self-integrate everything.
367
00:23:24,392 --> 00:23:27,452
It's painful, right? It's painful to integrate all these platforms.
368
00:23:27,832 --> 00:23:31,212
And what I find intriguing and interesting about where we are with cloud today
369
00:23:31,212 --> 00:23:36,792
is that everyone's pushing for at least the idea of these modular solutions
370
00:23:36,792 --> 00:23:40,992
where everyone has to have all these, the best. and I don't want vendor lock-in.
371
00:23:41,072 --> 00:23:42,512
I want to be able to swap things out.
372
00:23:42,712 --> 00:23:46,152
You're not going to do that. Like I look at it, I'm like, you're not going to do that.
373
00:23:47,012 --> 00:23:50,012
You're just not. Vendor lock-in is my least favorite term.
374
00:23:50,192 --> 00:23:54,572
Vendor lock-in is the funniest conversation I think I have because I'm just
375
00:23:54,572 --> 00:23:59,892
like, you're trying to say the problem we had in 2000 was vendor lock-in.
376
00:23:59,952 --> 00:24:00,892
That was not the problem.
377
00:24:00,972 --> 00:24:04,672
The problem was technology was not advanced enough to allow you to change.
378
00:24:04,912 --> 00:24:07,712
It's advanced enough now, regardless of what vendor you use,
379
00:24:07,752 --> 00:24:10,012
as long as it's cloud-based. It's actually pretty easy to move.
380
00:24:10,152 --> 00:24:14,472
I do love the idea of the consolidation that is starting to happen in the cloud
381
00:24:14,472 --> 00:24:17,852
where companies are getting smart about, all right, how do we do this?
382
00:24:17,932 --> 00:24:22,652
But they're trying to do it. Some do it well, smartly in terms of if I'm adding
383
00:24:22,652 --> 00:24:25,012
capabilities, it's built through our platform.
384
00:24:25,352 --> 00:24:29,252
Some people are acquiring tools and solutions and trying to integrate them in
385
00:24:29,252 --> 00:24:31,412
the cloud. And then it gets a little clunky and scary.
386
00:24:31,572 --> 00:24:35,812
We're now in a place where things are starting to get consolidated because of what you said.
387
00:24:35,992 --> 00:24:40,132
Look at the math. There's a billion little little dots on icons of companies
388
00:24:40,132 --> 00:24:44,652
on this giant capability map, and people are overwhelmed and confused.
389
00:24:44,912 --> 00:24:49,032
And I think Snowflake is and will always be a single SKU.
390
00:24:49,152 --> 00:24:52,952
When we make acquisitions, and when we build out net new features and capabilities.
391
00:24:53,432 --> 00:24:57,492
It's not something that you then have to turn around and pay for another license for.
392
00:24:57,592 --> 00:25:01,292
You buy your bucket of credits, and then you use them as you see fit.
393
00:25:01,412 --> 00:25:05,252
So back to your question about these decisions around LLMs and MLOps and and
394
00:25:05,252 --> 00:25:06,132
all that kind of stuff, right?
395
00:25:06,372 --> 00:25:10,072
It's it's for us, it's all about how are we driving that
396
00:25:10,511 --> 00:25:14,731
customer satisfaction in that NPS score, right? Like, we at Snowflake do not
397
00:25:14,731 --> 00:25:17,331
have a customer success org that does not exist at Snowflake.
398
00:25:17,471 --> 00:25:22,731
I didn't even, you know, I've worked with you guys since you worked with us years ago.
399
00:25:23,071 --> 00:25:27,971
And I didn't realize that this entire time. So that's yeah, yeah.
400
00:25:28,031 --> 00:25:32,851
And there's customer success office, like the idea of a customer success team
401
00:25:32,851 --> 00:25:34,911
not being there is support.
402
00:25:35,011 --> 00:25:38,411
Yes, success. No. And that's, that's an interesting differentiator,
403
00:25:38,471 --> 00:25:39,611
actually. so I appreciate that.
404
00:25:39,691 --> 00:25:44,171
I do want to pivot and give you the opportunity to continue the conversation
405
00:25:44,171 --> 00:25:46,511
we had over text regarding Gen AI.
406
00:25:46,811 --> 00:25:51,211
We have 15 minutes and I think it's important for us to cover this ground.
407
00:25:51,391 --> 00:25:55,591
When we were going back and forth, we were talking about the change in work, right?
408
00:25:55,671 --> 00:26:01,211
How is Gen AI actually going to impact work and maybe not just work for the
409
00:26:01,211 --> 00:26:03,831
general masses, but work for data teams?
410
00:26:04,031 --> 00:26:07,171
Yeah, so I want to go there with you. I'm curious of your opinion.
411
00:26:07,171 --> 00:26:12,691
I'm reading back through our texts to make sure I'm tracking to what I say.
412
00:26:13,051 --> 00:26:17,171
I think you've made a comment because people think that they're going to lose their jobs.
413
00:26:17,411 --> 00:26:22,311
Yes. But this is also what people who had tied their whole life to Oracle or
414
00:26:22,311 --> 00:26:27,911
Teradata, the DBAs of the world, felt when people started moving to the cloud.
415
00:26:28,251 --> 00:26:31,811
They pushed back really hard because they felt threatened. It is okay.
416
00:26:31,931 --> 00:26:33,491
It is okay to feel threatened.
417
00:26:33,651 --> 00:26:36,771
It is okay to feel like your job might be at risk. it's
418
00:26:36,771 --> 00:26:39,691
not okay to stop innovation because you don't want
419
00:26:39,691 --> 00:26:42,391
to change yourself right i think the the best advice i could
420
00:26:42,391 --> 00:26:45,231
give to to anybody in this space at this point is to be
421
00:26:45,231 --> 00:26:48,591
flexible in my own career right i started as a visualization guy
422
00:26:48,591 --> 00:26:51,491
i was a front-end bi analyst kind of person
423
00:26:51,491 --> 00:26:56,811
right and as the cloud became more prevalent the the bi end of the spectrum
424
00:26:56,811 --> 00:27:02,811
became less relevant and for me it it became important to diversify my skillset
425
00:27:02,811 --> 00:27:10,031
and be able to get an understanding of how ultimately do these BI products get fed with data?
426
00:27:10,367 --> 00:27:15,167
So I would say to anybody who is feeling threatened by generative AI that,
427
00:27:15,227 --> 00:27:17,987
but somebody's got to build the model. Somebody's got to tune the model.
428
00:27:18,207 --> 00:27:22,487
Somebody has to deploy the model. Somebody has to own the application that then
429
00:27:22,487 --> 00:27:24,427
the users interact with.
430
00:27:24,547 --> 00:27:27,227
If you feel like your job is going to be eliminated because you're not building
431
00:27:27,227 --> 00:27:33,227
ETL pipelines and you're not building dashboards anymore, then go take a course on LLMs.
432
00:27:33,287 --> 00:27:37,147
Go take a course on GPUs. Like, I understand that these are extremely technical
433
00:27:37,147 --> 00:27:40,667
constructs. And so it takes a lot to learn about them.
434
00:27:40,747 --> 00:27:45,827
If your other option is to just be a curmudgeon and not let innovation happen,
435
00:27:46,007 --> 00:27:50,847
because you're worried about your job, that's not ideal either.
436
00:27:50,947 --> 00:27:54,367
This these things will happen whether you get in the way or not.
437
00:27:54,367 --> 00:27:56,567
I'm going to print t-shirts curmudgeon.
438
00:27:57,007 --> 00:27:59,827
I totally agree with you.
439
00:27:59,907 --> 00:28:07,067
And I kind of mentioned this right during the first episode when I was a front
440
00:28:07,067 --> 00:28:11,547
end developer, I built BI solutions from scratch.
441
00:28:11,547 --> 00:28:14,587
And the pain and agony of
442
00:28:14,587 --> 00:28:17,827
seeing a what you see is what you get drag and
443
00:28:17,827 --> 00:28:21,887
drop lizzy wig bi platform in 2003
444
00:28:21,887 --> 00:28:28,067
i recalls when i first saw it from oracle yeah i almost had a heart attack because
445
00:28:28,067 --> 00:28:35,367
obi it was pre-obi it's kind of like it was it was the precursor to obi and
446
00:28:35,367 --> 00:28:39,087
i i still remember that i almost had a heart attack i didn't let anybody know
447
00:28:39,087 --> 00:28:41,687
that i was scared out of my mind and i I pivoted,
448
00:28:41,687 --> 00:28:47,487
I think the thing here is for people to realize that somebody has to design these solutions,
449
00:28:48,240 --> 00:28:51,340
you have to understand how these things are going to work. And you have to understand
450
00:28:51,340 --> 00:28:53,040
data quality is part of the problem.
451
00:28:53,360 --> 00:28:56,900
All the issues surrounding the work we do are still going to exist.
452
00:28:57,260 --> 00:29:00,480
It's just how we solve those issues are going to change. And you have to get
453
00:29:00,480 --> 00:29:03,900
uncomfortable more often now than we did in the past.
454
00:29:04,140 --> 00:29:10,740
I had a customer, he basically was like, so can I just train a model and get rid of ETL entirely?
455
00:29:11,280 --> 00:29:14,500
And I was like, no. I was like, Like in theory, sure.
456
00:29:14,700 --> 00:29:18,020
But is the cost of training that model going to be so great?
457
00:29:18,120 --> 00:29:21,420
And oh, by the way, is it going to perform like crap? Yes.
458
00:29:21,600 --> 00:29:24,720
So you're not going to get rid of ETL. And in the same way that,
459
00:29:24,720 --> 00:29:27,900
you know, Sandy, you and I had this debate over text about dashboards, right?
460
00:29:28,060 --> 00:29:33,720
I understand that the chat interface will make it easier for users to interact
461
00:29:33,720 --> 00:29:36,380
with the data in a way that is more exploratory.
462
00:29:36,600 --> 00:29:40,780
But what that's really going to get rid of is more of the Excel type of model
463
00:29:40,780 --> 00:29:44,480
of exploring and slicing and dicing data in certain ways.
464
00:29:44,600 --> 00:29:47,960
I pray to God that that happens. Your lips to God's ears, honestly.
465
00:29:48,040 --> 00:29:52,020
But there is still going to be a space for dashboard.
466
00:29:52,200 --> 00:29:54,660
It's not going to eliminate other ways of interacting with data,
467
00:29:54,760 --> 00:29:58,700
except for obviously in the more of the exploratory style, right?
468
00:29:58,780 --> 00:30:01,460
You're going to get a lot more people who are going to ask questions of data
469
00:30:01,460 --> 00:30:06,300
themselves and not go ask a BI analyst to go pull a report for them to answer
470
00:30:06,300 --> 00:30:08,720
one specific question. I think those days are gone.
471
00:30:08,940 --> 00:30:11,480
I think those days are, they need to be gone.
472
00:30:11,960 --> 00:30:17,240
I want everybody to move towards decision intelligence, where you're actually
473
00:30:17,240 --> 00:30:21,820
supporting decisions people have to make in the workflow in which they're making them.
474
00:30:22,300 --> 00:30:27,780
That's where I think this all needs to go towards. And the days of I go to a
475
00:30:27,780 --> 00:30:30,220
dashboard need to go away. way.
476
00:30:30,280 --> 00:30:34,480
I spent probably the first 10 years of my career doing nothing but front-end
477
00:30:34,480 --> 00:30:37,780
solutioning. And all I could think about is getting rid of it now.
478
00:30:37,940 --> 00:30:41,340
I'm just like, I'm so sick of it. I just want to help people make the right
479
00:30:41,340 --> 00:30:44,600
decision at the right time, in the right workflow, et cetera.
480
00:30:44,800 --> 00:30:47,240
I think that needs to start happening sooner rather than later,
481
00:30:47,300 --> 00:30:52,340
but there's so many barriers to that because BI teams are still working in isolation
482
00:30:52,340 --> 00:30:55,100
from the AI team, from the digital team.
483
00:30:55,360 --> 00:31:02,140
I think I understand where you're coming from, But I think that the dashboard
484
00:31:02,140 --> 00:31:04,500
paradigm is never going to go away, right?
485
00:31:04,620 --> 00:31:09,100
And even if the dashboard paradigm shifts to being basically,
486
00:31:09,160 --> 00:31:11,520
how do I embed KPIs into your workflow?
487
00:31:11,740 --> 00:31:15,740
That still has a dashboard-like feel, right?
488
00:31:15,900 --> 00:31:18,880
And part of our debate over text was around operational, right?
489
00:31:18,940 --> 00:31:21,420
The operational dashboards will continue to exist, period.
490
00:31:21,740 --> 00:31:26,820
That's just how it's going to work, right? So I get decision intelligence and
491
00:31:26,820 --> 00:31:32,000
I get making that point. But like, when it comes to a user interacting with
492
00:31:32,000 --> 00:31:36,180
data, you have to make sure that it fits in terms of their own maturity.
493
00:31:37,429 --> 00:31:43,089
Putting it all in chatbots is good, except for the users who don't know how
494
00:31:43,089 --> 00:31:47,009
to ask questions, who just want the specific answer to the specific question
495
00:31:47,009 --> 00:31:50,289
that they ask themselves every day to do their job. It's that bad.
496
00:31:51,129 --> 00:31:56,269
You don't know how to ask questions? Wow. I mean, come on.
497
00:31:56,309 --> 00:32:01,229
You and I have spent enough time with enough clients in this world who ask the worst questions.
498
00:32:02,249 --> 00:32:07,129
Let's be clear. here. I plead the fifth on that one. I am not subscribing to that, James.
499
00:32:07,489 --> 00:32:10,329
Well, you still have clients to deal with. I get to be off the center side.
500
00:32:10,449 --> 00:32:12,969
I don't have to do that. There are no dumb questions.
501
00:32:13,689 --> 00:32:15,809
Well, I do tell people there's no such thing as a dumb question,
502
00:32:15,869 --> 00:32:19,069
only a dumb answer. But it doesn't mean you don't. It doesn't mean you know
503
00:32:19,069 --> 00:32:20,049
how to ask the question, right?
504
00:32:20,249 --> 00:32:24,309
I mean, that applies to to generative AI as well, right?
505
00:32:24,409 --> 00:32:29,029
I mean, there are dumb answers that come back from from these models as well.
506
00:32:29,089 --> 00:32:31,849
We have to be able to one, except you're going
507
00:32:31,849 --> 00:32:34,749
to get a dumb answer but to put guardrails in
508
00:32:34,749 --> 00:32:37,569
place the human in the loop to to make
509
00:32:37,569 --> 00:32:40,729
the decision around whether or not that's the answer we can actually use
510
00:32:40,729 --> 00:32:46,009
and trust yeah i could not agree with that statement more like i as much as
511
00:32:46,009 --> 00:32:49,769
as much as ml and this idea of prescriptive analytics in terms of like actually
512
00:32:49,769 --> 00:32:53,729
letting the computer make decisions i like there's a world where i understand
513
00:32:53,729 --> 00:33:00,269
why that that that happens so like google maps right nobody's in nobody is sitting at the terminal.
514
00:33:00,989 --> 00:33:03,949
Seeing all the google maps requests in and then telling them
515
00:33:03,949 --> 00:33:06,709
which directions to go right a
516
00:33:06,709 --> 00:33:09,849
computer is making that decision i i get that and guess
517
00:33:09,849 --> 00:33:12,749
what google maps does it wrong a lot and so
518
00:33:12,749 --> 00:33:16,129
you have to you as the user has to decide i trust
519
00:33:16,129 --> 00:33:18,949
this or no i really don't so we were we
520
00:33:18,949 --> 00:33:21,749
went up to north my my family and i went up to northern maine for
521
00:33:21,749 --> 00:33:24,789
the eclipse last week it was beautiful it was it
522
00:33:24,789 --> 00:33:27,469
was a beautiful day it was the coolest experience i've ever had but
523
00:33:27,469 --> 00:33:30,929
it took us what should have been a at most five
524
00:33:30,929 --> 00:33:33,589
hour drive took eight hours to get home google maps just like
525
00:33:33,589 --> 00:33:36,569
didn't work and everybody ended up on the the area at
526
00:33:36,569 --> 00:33:39,489
franconia where 93 and three come together and they went
527
00:33:39,489 --> 00:33:42,429
five miles in five hours i i i
528
00:33:42,429 --> 00:33:45,629
agree i think it was we can find instances where
529
00:33:45,629 --> 00:33:48,789
it's not going to work when there's an event that is so mass
530
00:33:48,789 --> 00:33:51,469
in terms of the number of people jumping on the highway and the
531
00:33:51,469 --> 00:33:54,509
information doesn't get back fast enough for it to update its
532
00:33:54,509 --> 00:33:57,129
algorithm yeah of course but i
533
00:33:57,129 --> 00:33:59,969
but i i looked at the map and i said i don't
534
00:33:59,969 --> 00:34:04,789
like what this route is and i had to go manually put in a point on the map to
535
00:34:04,789 --> 00:34:10,609
force google maps to take me in that direction right so like that's that's sort
536
00:34:10,609 --> 00:34:14,369
of the manual intervention that you're talking about anjali of like i as a user
537
00:34:14,369 --> 00:34:18,869
have to be smart enough and say this doesn't make any sense Generally,
538
00:34:18,889 --> 00:34:22,869
I might get rid of some level of IT jobs in theory.
539
00:34:22,989 --> 00:34:25,569
Yeah, I don't think the jobs are going to go away. I think they're just not
540
00:34:25,569 --> 00:34:28,709
going to scale the way they've been scaling. Sure.
541
00:34:29,029 --> 00:34:31,669
That's kind of where I'm at. The scalability is going down a bit.
542
00:34:31,669 --> 00:34:35,909
Like you don't have to have thousands of data engineers if you're a data heavy company.
543
00:34:36,321 --> 00:34:39,481
That's going to probably simmer down a bit. You'll be able to do a lot more
544
00:34:39,481 --> 00:34:42,961
with less. So I don't see anything completely disappearing.
545
00:34:43,221 --> 00:34:49,921
However, I will say the logo for their podcast, a logo for a friend's Facebook group.
546
00:34:50,001 --> 00:34:53,221
She was pinging me yesterday, just two days ago, asking for a logo.
547
00:34:53,441 --> 00:34:57,021
Hey, you did this AI thing for your podcast logo. Can you go do one for me?
548
00:34:57,201 --> 00:35:00,041
And it was like around the barrel, I think it's called. She's like,
549
00:35:00,061 --> 00:35:01,901
it's a new Facebook, but I'm trying to do it. I was like, fine.
550
00:35:01,961 --> 00:35:03,901
So I literally opened up my phone.
551
00:35:04,001 --> 00:35:06,441
I didn't even use my computer. I had chat GPT on my phone.
552
00:35:06,581 --> 00:35:11,061
I asked it and within the third hit, it had it. Five minutes later, she's got a logo.
553
00:35:11,301 --> 00:35:16,221
And so I feel bad for all the artists out there on Fiverr that was creating
554
00:35:16,221 --> 00:35:19,901
logos for five bucks or a hundred bucks a pop. That industry is gone.
555
00:35:20,081 --> 00:35:26,601
So apologies to them. But I think that's going to be the tough one for us.
556
00:35:26,781 --> 00:35:31,081
I do have to wrap this up. I have a client call that I had scheduled after this,
557
00:35:31,101 --> 00:35:32,521
unfortunately, so I got to run over there.
558
00:35:32,601 --> 00:35:36,721
But James, James, what is your last piece of advice to anybody who's scared
559
00:35:36,721 --> 00:35:40,181
to death in terms of, I'm a data engineer, I'm just starting my career.
560
00:35:40,381 --> 00:35:42,161
What should I focus on? Learn Python.
561
00:35:42,461 --> 00:35:45,441
There's a lot of people who will tell you, oh no, SQL will never go away.
562
00:35:45,581 --> 00:35:46,861
Yeah. They're not correct.
563
00:35:47,141 --> 00:35:51,961
Python is, from a declarative engineering perspective, Python is where people are moving.
564
00:35:52,361 --> 00:35:56,441
And Python is super flexible. I said earlier, being flexible is the most important
565
00:35:56,441 --> 00:35:57,421
thing that you can do for yourself.
566
00:35:57,661 --> 00:36:00,741
And I think, at least in terms of what's happening in the next five years,
567
00:36:00,981 --> 00:36:05,641
having an understanding of Python is is going to allow you to do a lot of things
568
00:36:05,641 --> 00:36:07,381
and be and help be successful.
569
00:36:07,621 --> 00:36:12,141
So if I had any advice for a budding data engineer, the first thing I would
570
00:36:12,141 --> 00:36:14,181
tell you is to go learn Python, learn Python.
571
00:36:14,421 --> 00:36:16,881
I love it. Well, thank you, James, for being on.
572
00:36:17,181 --> 00:36:20,861
Of course. Thank you for having me. I mean, it was exactly what I expected.
573
00:36:20,981 --> 00:36:23,221
I expected this from you. So I appreciate that.
574
00:36:23,361 --> 00:36:27,821
And we probably need to have a round two in 10 years to figure out was I right
575
00:36:27,821 --> 00:36:29,481
about operational dashboards?
576
00:36:29,561 --> 00:36:32,021
Or was James's right about operational dashboards?
577
00:36:32,161 --> 00:36:35,981
I'm putting the that down now. I'll Venmo the money to you and let's do it.
578
00:36:36,041 --> 00:36:40,141
Done. Thank you. Well, have a great weekend. Bye, Anjali. I'm off.
579
00:36:40,400 --> 00:37:08,668
Music.